Review of the AGRIS paper : Discovering, Indexing and Interlinking Information Resources
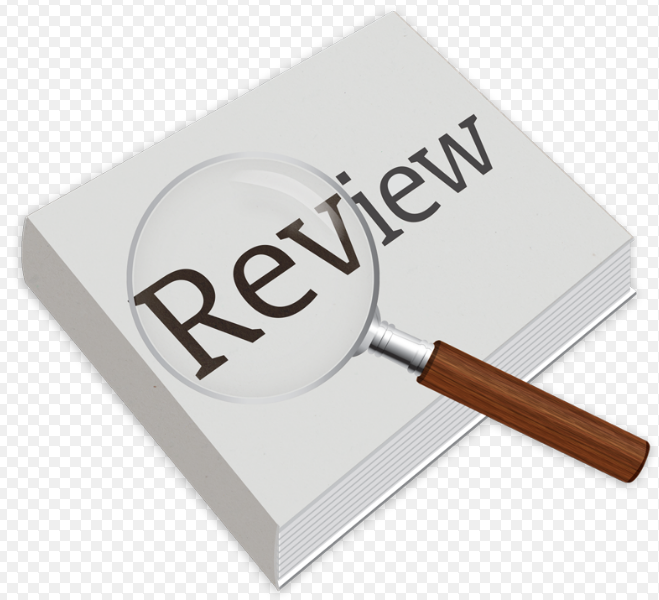
This is a summary review of the AGRIS paper entitled, Discovering, Indexing and Interlinking Information Resources, which was published in the Open knowledge in agricultural development channel.
Objective
This paper discusses an evaluation study on a benchmark sample of AGRIS articles carried in order to determine the relevance between crawled web resources and the AGRIS database.
Information systems that facilitate access to scientific literature must learn to cope with this valuable and varied data, evolving to make this research easily discoverable and available to end users. The paper describes the incremental process of discovering web resources in the domain of agricultural science and technology.
Background
Social media has affected the scientific world, as has the internet itself. Scientists now share their research interests, theories and outcomes across numerous channels, such as personal blogs, other thematic web spaces where ideas, activities and partial results are discussed.
Meanwhile, AGRIS is the International System for Agricultural Science and Technology, a collection of nearly 8 million multilingual bibliographic resources spanning the last forty years and produced by a network of more than 150 institutions from 65 countries. Some AGRIS data sources are unique to the system and AGRIS is the only way in which they can be accessed.External resources available in AGRIS mashup pages are not only bibliographic metadata, but also distribution maps, statistics, germplasm accessions, and so on.
In this paper we explore a new data source available in AGRIS mashup pages: the web itself. The challenge is that this information is usually not exposed using web services that can be consumed by machines, and the only way to access this rich amount of data is to use web search engines that typically return thousands of results, largely meaningless. In addition, most blogs and websites are not well categorized and so it is difficult for users and machines to discover what is actually relevant to the topic of interest.
Methodology
The paper discusses the crawling and analysing web resources to populate our “Crawler Database”; a SPARQL endpoint with AGROVOC annotations of web resources identified by the URL from which they were crawled. By providing web resources with semantics we can use the AGROVOC descriptions of AGRIS bibliographic entries to interlink AGRIS and the Crawler Database.
This linking is then exploited by a recommender that identifies web resources that are relevant to AGRIS entries. Furthermore, we also discuss the preliminary testing of the SemaGrow Stack as the computational infrastructure for interlinking the AGRIS bibliographic database with the Crawler Database. The query federation and data integration functionalities of the SemaGrow Stack facilitate setting up experiments aiming at estimating semantic similarity between AGRIS entries and other resources. We computed the precision of recommendations considered as “relevant” by our algorithm, commenting on some possible improvements to the process used and described in our work.
Outcomes of our evaluation study are presented in the “Analysis of relevance” section, together with a new picture displaying the cumulative distribution of AGRIS records over the number of relevant recommendations. Furthermore, we created a separate section “Analyzing the algorithm performance” where we compared the execution time of the recommender system in both the “individual” and “federated” modes.
The section “The output of the recommender system” was removed, since it contained only a sample RDF/XML fragment that was not very significant. Lastly, the definition of the custom algorithm was removed and minor improvements have been made to the text, as suggested by reviewers.
The results
This experiment(s) led directly to the addition of a new data source to the AGRIS mashup pages: the dataset of related resources crawled from the web. The web contains much latent knowledge, especially when that knowledge is expressed as unstructured and poorly categorized full-text content.