Implement effective Open Data & Keep it alive with Open Data TOOLKIT
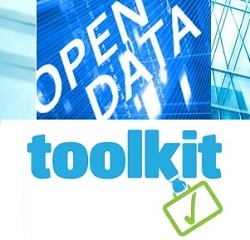
“Around the world, a movement called the “OPEN DATA revolution” is under way to make data available for public use. This movement is expected to generate new insights, drive better decision-making, and enable governments, civil society, and the private sector to better target interventions and programs”, - Open Data Revolution to Fight Global Hunger, USDA, 2017.
In March, 2017, the European Data Portal released The majority of the EU28+ countries have successfully developed a basic approach to address Open Data, but are at the same time struggling with a number of barriers (related and dependent on each other), hindering them to move forward with Open Data... |
"Ask not what you can do for Open Data; ask what Open Data can do for you" (by Jack Leeming | naturejobs |19 Jun 2017), how can we better pin down different facets of Open Data (OD), and make them happen.
Continuing interest and debate about FAIR and Open Data has triggered rather extensive international efforts to develop recommendations and services that may be helpful to have greater exploratory research, constructive discussions and solutions based on interoperable data.
The resources listed below are not ‘cumulative’, but are rather related (even if independent from one another), and may be implemented in any combination, incrementally, to increase degrees of FAIR-ness of your data environment (i.e. Findability, Accessibility, Interoperability, and Reusability).
It is also important to be aware of the fact that 'GOOD DATA' should be linked to a set of ‘BENEFITS’. According to The Data on the Web Best Practice (W3C, 2017), - each benefit represents an improvement in the way how datasets are available on the Web:
Comprehension (human) | Processability | Discoverability | Reuse |
Trust | Linkability | Access | Interoperability |
WHAT ? | WHY ? HOW ? |
Understanding OD Basics |
|
Feasibility of OD & Data Management |
|
OD Strategy : a Vision, Mission, measurable Goals & an Action Plan |
|
OD & Research Data Management Policy : a Guiding Framework for Data Management |
*** Although a policy is a good starting point for strong OD practice, policy implementation must be supported by capacity building, leadership, clear communication and a mixture of incentives to make the process of culture transformation possible (GODAN). |
OD & |
|
Data Management Plans (to be continuously maintained and kept up-to-date) & Data Analysis |
|
Measures & Tools for OD Quality & Trust |
*** Having shared policies and standards in place that set out what best practice data publishing looks like, and how it will be monitored and assessed, - is the necessary backbone for any potential hard levers enforcing data quality. |
Data-cleaning Tools
|
|
Data Collection |
|
Ethical issues, Licensing |
*** Having the machine-readable licence including a complete description of the metadata is important for your content and data to be correctly harvested by machines, e.g. search engines and web APIs. Licence metadata should point to a URI or at least a URL of a published licence. |
Responsible OD | |
Data Deposition | |
Data citation (Meta)Data linked to Persistent Identifiers / PIDs = long-term machine-Actionable (Meta)Data A Data Object = a Data Item
|
***Regarding PIDs, the most common practices are to use: *** Where a data publisher is unable or unwilling to manage its URI space directly for persistence, an alternative approach is to use a redirection service such as purl.org'. *** Make data available in a machine-readable standardised data format that is easily parsable including but not limited to CSV, XML, Turtle, NetCDF, JSON and RDF. *** Data without metadata cannot be understood by machines, i.e. a metadata element gives meaning to the data. *** The FAIR principles foresee that dataset metadata are registered in dataset catalogues where they can be more easily found. |
Data Curation Data Stewardship |
|
Data Publishing & Visualisation |
|
Interoperability, Data Sharing & Re-use
NOTE: Implementing all the technical requirements can In most cases, rather than developing ad hoc software, it may be very convenient to use existing tools. Evaluate them against all the criteria depending on the specific data sharing needs. Make informed decisions about your data environment.
|
*** The interoperability of data is achieved through the interoperability of metadata (The Data on the Web Best Practice, W3C, 2017). *** Architectural interoperability: is related to higher-level data exchange protocols designed for (meta)data sharing. *** Structural interoperability: defines the syntax of the data exchange through data formats and data structures. This is the level where (meta)data become machine-readable. *** Semantic interoperability takes advantage of both the structuring of the data exchange and the codification of the data with help of vocabulary(ies) to interpret the data. *** (Meta)data have to be not just readable but ‘parsable’ by machines. Since ‘parsing’ = splitting a file or other input into pieces of data that can be easily stored or manipulated, - the more regular and rigorous a format is (e.g. CSV on the Web, XML and JSON), the easier it is to parse it. *** URIs are the ‘glue’ of RDF triples. RDF grammar has been successfully applied to XML: RDF XML Specification. *** The most popular protocols for exposing data as a service (DaaS) are OAI-PMH, SPARQL, RESTful APIs. *** Use persistent URIs as identifiers within datasets : datasets should use and reuse other people's URIs as identifiers where possible. See: W3C. Best Practices for Publishing Linked Data. See also: JSON for Linking Data.
|
Monitor Success of Data & Metadata : Data Objects should be persistent, with emphasis on their metadata. |
*** Consider implementing metrics to your data in order to evaluate its performance and success, through several indicators. Engage re-users: improve your work with datasets by acting on their feedback. |
Communicating Value & Impact of OD Create a thriving ecosystem of data Re-Users, Coders, and application developers ! |
|
“Ready-to-use” Toolkits for OD & Data Management |
|
Training & Guides on OD &
|
|
The essence of identifying and sharing good practices is to learn from others and to re-use knowledge. The biggest benefit consists in well-developed processes based on accumulated and shared experience ... If you are aware of other resources that could be added to the list above, please:
Collective Knowledge unleashes our Collective Power ! |
P.S. You might be also interested in checking out some contributions from the international community to outline the future directions of OD.
To keep up-to-date with AIMS news, please, Sign up for AIMS news; keep up to date via our Twitter feed at @AIMS_Community.
And, thanks again for your interest !