Put FAIR principles into practice and enjoy your data!
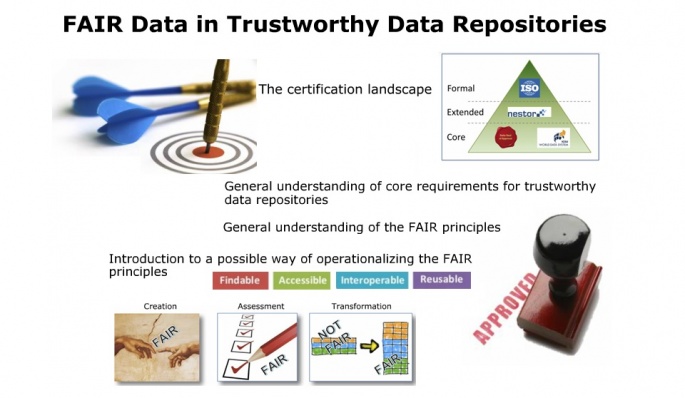
There is a growing demand for quality criteria for research datasets stored, managed and shared in reliable and trustworthy way. The FAIR Data in Trustworthy Data Repositories Webinar - held on 12-13 December, 2016 - shed light on FAIR principles, Trustworthy Digital Repositories and a possible way of operationalizing the FAIR principles.
During the webinar, Dr Peter Doorn (from Data Archiving and Networked Services: DANS) and Dr Ingrid Dillo (DANS) presented and compared the two sets of principles and discussed their tangible operationalization. The presenters would highly appreciate feedback from the audience on the ideas regarding matching between FAIR principles and Trustworthy Digital Repositories. The Webinar was co-organised by DANS, EUDAT and OpenAIRE. |
Many might have a question: Everybody wants to play FAIR research, but how do we put the principles into practice?
There is a growing demand for quality criteria for research datasets. A number of approaches and recommendation to tackling this issue are proposed by DSA (Data Seal of Approval for data repositories) and FAIR (Findable, Accessible, Interoperable and Reusable) principles. These two recommendations do not make value judgements about the content of datasets, but rather qualify the fitness for data reuse in an impartial and measurable way.
By bringing the ideas of the DSA and FAIR together - that get as close as possible to giving quality criteria for research data - you will be also able to perform an operationalization of DSA & FAIR principles in any certified Trustworthy Digital Repository.
FAIR Guiding Principles versus DSA
In 2014 the FAIR Guiding Principles were formulated. The well-chosen FAIR acronym is highly attractive: it is one of these ideas that almost automatically get stuck in your mind once you have heard it. In a relatively short term, the FAIR data principles have been adopted by many stakeholder groups, including research funders.
The FAIR principles are remarkably similar to the underlying principles of the aforementioned DSA (2005) that asserts that the reliable data:
- can be found on the Internet,
- are accessible (clear rights and licenses),
- are in a usable format [there is no universal agreement, general exists],
- are identified in a unique and persistent way so that they can be referred to.
Essentially, the DSA presents quality criteria for digital repositories, whereas the FAIR principles target individual datasets.
***
Opening the webinar, Dr Peter Doorn briefly introduced:
1. DANS Services that promotes sustained access to digital research data. DANS developed the DSA (Data Seal of Approval for data repositories). At the moment over 60 seals acquired around the globe, but with the focus on Europe.
2. NARCIS - National Academic Research and Collaborations Information System. The metadata of the publications and datasets within NARCIS, such as title or author's name, can be reused in other services (18 services currently harvest the aggregated publications within NARCIS).
3. DANS EASY portal where research data can be deposited, searched and retrieved. The EASY digital archive is assessed by NESTOR Seal 2016 and the DSA (Data Seal of Approval for data repositories).
4. The Trusted Repositories Audit & Certification: Criteria and Checklist (TRAC) evaluation tool.
On top of the core/basic certification provided by the Data Seal of Approval/DSA (that is easy to assess repositories) and ISCU World Data System/WDS, the NESTOR seal grants an extended certification. While the NESTOR seal can be obtained as a standalone solution, it also fits into the European Framework for Audit and Certification. On the top of the NESTOR there is a formal audit and certification provided by ISO. |
NOTE: while # the DSA and WDS are lightweight tools (you need not to comply with all their requirements) that can be easily used for self-assessment by the community, the assessment and certification with # ISO [that is much deeper than DSA, WDS and NESTOR] requires compliance with numerous regulatory and legal requirements as well as periodic re-assessment audits to confirm that the organization/service remains in compliance with all requirements of the standard. |
Among DANS partners there is also the Research Data Alliance (RDA). This partnership stands out as being particularly important to realize efficiencies of and simplify assessment options, stimulate more certifications, increase impact on the research community. The main outcome of these two years-partnership (the RDA-DSA-WDS Partnership on Repository Certification Working Group) is the DSA-WDS common catalogue of requirements for core repository assessment with 16 requirements, distributed as follows:
- Organizational infrastructure (with 6 requirements);
- Digital Object management* (with 8 requirements);
- Technology (with 2 requirements);
- Additional information and applicant feed-back (with 2 requirements).
* Digital Object (here) = an Identifiable Data Item with Data elements + Metadata + an Identifier
All requirements are accompanied by text, thus providing context with a lot of additional information useful for assessment of digital repositories.
On the 24 November, 2016, WDS And DSA announced Unified Requirements for Core Certification of Trustworthy Data Repositories developed through the RDA DSA–WDS Partnership Working Group. This also means that common procedures for assessment will be supported by shared testbed for assessment.
DSA and FAIR principles: what matches?
DSA Principles - for data repositories | FAIR (Findable, Accessible, Interoperable, Reusable) data - both for machines and for people |
The data can be found on internet | FINDABLE |
The data are accessible (clear rights and licenses) | ACCESSIBLE |
The data are in a usable format | INTEROPERABLE |
The data are reliable | REUSABLE |
The data are identified in a unique and persistent way to be referred to | (CITABLE) |
The FAIR Guiding Principles for scientific data management and stewardship are clearly described by an article published (2016) on the SCIENTIC DATA portal.
It is worth noting that the FAIR principles have been designed with these research workflow steps and concerns in mind
FINDABLE (F) | or discoverable, data and metadata should be richly described to enable attribute-based search |
broadly ACCESSIBLE (A) | data and metadata should be retrievable in a variety of formats that are sensible to humans and machines using persistent identifiers (PID) |
INTEROPERABLE (I)
| the description of metadata elements should follow community guidelines that use an open, well defined vocabulary |
REUSABLE (R) | the description of essential, recommended, and optional metadata elements should be machine processable and verifiable, use should be easy and data should be citable to sustain data sharing and recognize the value of data. |
The FAIR principles can be implemented for different aims:
FAIR data management | Posing requirements for new data creation |
FAIR data assessment | Establishing the profile of existing data |
FAIR data technologies | Transformation tools to make data FAIR |
FAIR Principles are also supported and promoted by HORIZON 2020 Programme, in particular, by the Guidelines on FAIR Data Management in Horizon 2020 including 16 + 23 = 39 questions for assessment!..
Considering growing demand for simplification of quality criteria for research datasets and a way to assess their fitness for use, the presenters of the webinar have invited interested parties to:
- combine DSA & FAIR, as quality criteria for: (i) digital repositories, through the DSA core certification; (ii) research data (sets), by means of FAIR principle;
- operationalize the principles, which are easily implementable in any trustworthy digital repository (TDR).
The core DSA certification requirements and the FAIR principles form a perfect couple for quality assessment of research data and TDR.
DANS is currently developing an operationalization of these principles, taking into consideration that:
- Data archive staff will assess FAIRness of data upon ingest;
- Data users will assess FAIRness of data upon reuse;
- Scoring mechanism should be as automatic as possible.
WHAT IS SCORING MECHANISM?
EACH DATASET - A FAIR PROFILE:
Work on further explanation (more detailed, examples) on how to operationalize the principles and provide support for FAIR data assessors/reviewers is in progress…
In particular, DANS is going to develop an independent FAIR assessment website - like the DSA-website, to which repositories will link to make FAIR data assessment, as automatic as possible. In particular, this website will provide:
Assessment tool (“questionnaire” with explanation and examples for each criterion); Online database containing: - Repository holding the dataset; - PID (+basic metadata such as name) of datasets; - Review info (ID can be withheld – anonymous reviews should be possible); - FAIR profile and scores; Analytics of FAIR profiles. |
Ideally: all certified trustworthy repositories should contain FAIR data.
But: the FAIR scoring mechanism is applicable in any repository.
All in all: we need quick and effective methods to access quality of data and repositories.
Let’s start small.
Check a series of workshops which took place during 12th International Digital Curation Conference, Edinburg, 20-23 February, 2017:
- How EUDAT Services could support FAIR data
- OpenAIRE services and tools for Open Research Data in H2020
- Essentials 4 Data Support: the Train-the Trainer version
In case you missed the live webinar, you can still take the most out of this event as the material is available below:
· the slides presented in the webinar: click here to view the presentation.
· the recording of the webinar: click here to view the video.
· the chat log (in case you would like to consult some of the links shared during the webinar): click here to browse the document.
|
Check out to learn more:
- Recommended-formats (UK Data Services)
- Information about depositing data (DANS)
Legal Interoperability of Research Data: Principles and Implementation Guidelines (ZENODO)
- Information that you should familiarize yourself with before using Creative Commons
- Data FAIRport initiative
- Write a Data Management Plan [it is not important to answer all questions in the DMP]
- An EUDAT-based FAIR Data Approach for Data Interoperability
- Trust and Certification (EUDAT Webinar)
- Are the FAIR Data Principles Fair? (LIBER Webinar recording)
- LEARN (LEaders Activating Research Networks) (project)
- Audit and certification (Digital Preservation Coalition)
- TrustedDigitalRepository.eu
- Preserving digital heritage: At the crossroads of Trust and Linked Open Data (IFLA article)
- Guidelines on FAIR Data Management in Horizon 2020 (AIMS blog post)
- FAIR Data Management. Towards the new era of Open Science (AIMS blog post)
- Responsible Data Science. Ensuring Fairness, Accuracy, Confidentially, Transparency (FACT) (AIMS blog post)
- LICENTIA: a web site to Choose the License for your Data (AIMS blog post)