FAIR Principles & Digital Objects: The role of METADATA
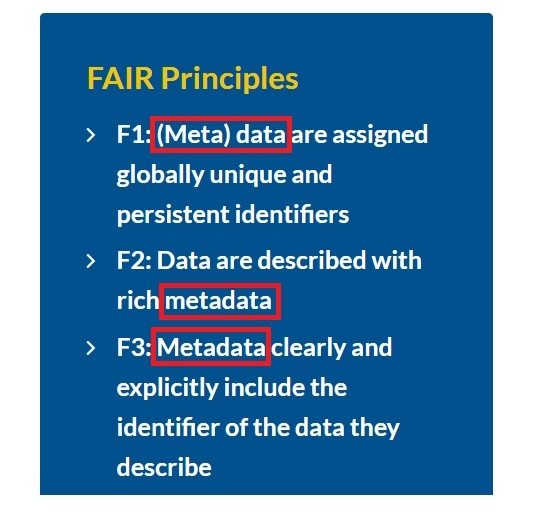
The advancement of digital science thrives on the timely sharing and accessibility of digital data, and every day research activities provide unlimited amounts of data that could have an impact on achieving the objectives of a number of seamless research processes and their quality.
With all the information and data available, it is important that your data are valuable, properly described and managed. By taking care of these matters at an early stage, your research will stand out and foster new promising integrative scientific approaches and studies based on FAIR Data.
Image source: CGIAR
FAIR Data Principles (Findable, Accessible, Interoperable, Reusable) aim to help you create, share and re-use quality, valuable, and responsible data. Only by leveraging and applying the evidence about FAIR data impact we can help data live up to its long-term potential.
FAIR DATA PRINCIPLES: BACKGROUND & EXPLANATION
|
FAIR PRINCIPLES & DIGITAL OBJECTS: THE ROLE OF METADATA
Metadata – or ‘data about data’ – is arguably one of the most powerful tools available in scholarly communications. Good metadata enables discoverability and access, and (potentially) eliminates errors.
Interoperable metadata linkages and promoting data citations could provide an efficient model or a framework enabling access, management and re-use of any Digital Object (D.O.) – for example, a data set or journal paper - in a long-term.
"Implementing FAIR requires a model for #FAIRData Objects which have a PID linked to different types of essential metadata, including provenance and licencing. Use of community standards and sharing of code is fundamental for interoperability and reuse." (Twitter)
According to the FAIR Principles, to be(come):
| D.O./DATA | (META)DATA |
F | FINDABLE,D.O./data | ... sufficiently rich metadata and a unique and persistent identifier using a standardized communications protocol. Metadata should clearly and explicitly include the identifier of the data it describes [check the FAO Meaningful Bibliographic Metadata (M2B) recommendations: IDENTIFIER] |
A | ACCESSIBLE,D.O./data | ... metadata understandable to humans and machines. This metadata is registered or indexed in a searchable resource and deposited in a trusted repository. (Meta)data should be supported by open, free, and universally implementable protocols which allow for an authentication and authorization procedure, where necessary. Metadata is accessible, even when the data is no longer available |
I | INTEROPERABLE,D.O./data | ... metadata that use a formal, accessible, shared, and broadly applicable language for knowledge representation [check the FAO LODE-BD: encoding strategies for producing meaningful Linked Open Data (LOD)-enabled bibliographical data]. (Meta)data should meet domain relevant community standards [check: FAIRsharing, AgriSemantic Map of standards, Basel Register of Thesauri, Ontologies & Classifications]. (Meta)data includes qualified references to other (meta)data. |
R | REUSABLE,D.O./data | ... metadata with clear usage licenses and provide accurate information on provenance. (Meta)data is richly described with a plurality of accurate and relevant attributes, and meets domain-relevant community standards. |
THE NEED FOR MACHINE ACTIONABLE METADATA
Machine-actionable metadata (supported by Machine-actionable Data Management Plans) are core to the FAIR Principles.
GO FAIR and RDA (Research Data Alliance) members have launched the 'Metadata for Machines' workshop series (M4M) to assess the state of metadata practices in data-related communities and stimulate the creation and re-use of FAIR metadata standards and machine-ready metadata templates (definitions of metadata categories).
Collectively, the M4M series of workshops result in recommendations about metadata and an Open repository of machine-ready, easy to use and interoperable FAIR metadata templates and components. Anyone can access this ’sea’ of metadata templates/components, re-use them as they see fit, and deploy them using metadata editors and other data capture tools (Learn more from: GO FAIR, Making it easy for humans to make metadata for machines).
HOW TO MAKE DATA BE(COME) FAIR TOGETHER?
'If you want to go fast, go alone. If you want to go far, go together!' (African Proverb)...
PLAN - check, e.g. - DSW: Data Stewardship Wizard | CREATE -check, e.g. - FAIRifier
| PUBLISH - check, e.g. - FAIR Data Point
| FIND - check, e.g. - FAIR Data: Many paths lead to the EOSC |
ANNOTATE - check, e.g. - ORKA (Open Reusable Knowledge Annotator) and other tools in FAIR Data tools indexed in Dutch Techcentre for Life Sciences | EVALUATE - check, e.g. - FAIR Metrics Framework; - check, e.g. - FAIR self-assessment tool | METADATA - check, e.g. - RDA METADATA Catalog - check, e.g. - CEDAR (CENTER for EXPANDED ANNOTATION and RETRIEVAL) | STANDARDS - check, e.g. - FAIRsharing.org
|
Joint implementation based on FAIR Principles for research data, algorithms, processes, software etc. (check: Research Data Alliance outputs and their adoption stories and adoption use-cases):
- stimulates cooperation, convergence and global (data) interoperability;
Discover RDA IGAD ‘Landscaping the Use of Semantics to Enhance the Interoperability of Agricultural Data' … and keep coping with FAIRifying challenge! |
- prevents further fragmentation and enhance opportunity to help shape the Internet of common/shared FAIR Data and Services;
- pushes 'increasingly speaking with one voice' (check, e.g. GO FAIR Implementation Networks).
FAIR DATA-RELATED
- GO FAIR Processes & Implementation Networks (INs). Towards Food Systems IN
- Cost-benefit analysis for FAIR research data Policy recommendations - EU Study
- Turning FAIR into reality. Final report and action plan from the European Commission expert group on FAIR data
- Interoperability in practice and FAIR data principles
- RESPONSIBLE DATA GUIDELINES: Managing Privacy and Personally Identifiable Information
- RDA FAIR Data Maturity Model Working Group Case Statement
- Common Patterns in Revolutionary Infrastructures and Data
- FAIR Webinar series : #1 Findable #2 Accessible #3 Interoperable #4 Reusable
- Enabling FAIR Data Project (COPDESS: Coalition on Publishing Data in the Earth and Space Sciences)
- Enabling FAIR Data Across the Earth and Space Sciences
- FAIRsharing: working with and for the community to describe and link data standards, repositories and policies, by Sansone et al., in BioRxiv, Preprint (2018)
- Put FAIR principles into practice and enjoy your data!
- From the Top 10 FAIR Data Things Global Sprint. Take a look at dedicated GITHUB space
- FAIR data: What and Why? Easier said than implemented?
- Make FAIR your Datasets using Springer Nature RESEARCH DATA SUPPORT
- AgBioData ensures Standards and Best Practices for acquisition, display and retrieval of Genomic, Genetic & Breeding Data
- METADATA 2020 : details and crosswalks of the recommendations. Can we agree?
You are invited to Sign up for AIMS News, follow @AIMS_Community on Twitter... And, thanks again for your interest!