Open & Big Data : shifts in roles, power relations, quality and knowledge integration
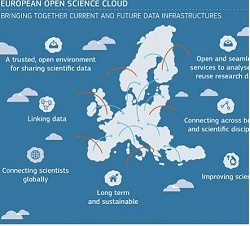
Image sources: (1) The Agricultural Model Intercomparison and Improvement Project (AgMIP; the NextGen strategy encouraging the development of NextGen data, models, and knowledge tools to support decision makers); (2) OpenData Nederlands; (3) Forbes(*)
(*) “There are hundreds (if not thousands) of free data sets available, ready to be used and analyzed by anyone willing to look for them… Data is ubiquitous - but sometimes it can be hard to see the forest for the trees, as it were…”
While Open Data are publicly available (under licences) data for anyone to use and reuse, Big Data refers to very large, complex, rapidly-changing datasets. Open Data brings a perspective that can make Big Data more transparent, useful, to make data-driven decisions more participative, and to solve complex problems. Both Open & Big Data can transform our operational world, but when government turns Big Data into Open Data it's especially powerful. |
OPEN & BIG DATA : USE & SIZE : WHERE & HOW DO THEY OVERLAP?
“Around the world, a movement called the “open data revolution” is under way to make data available for public use. This movement is expected to generate new insights, drive better decision-making, and enable governments, civil society, and the private sector to better target interventions and programs”, - Open Data Revolution to Fight Global Hunger, USDA, 2017.
On its turn, Big Data can help analyze patterns and trends, as well as raise the complex issues and empower communities of practice within different parts of the value chain (How GODAN Wants to Empower the World’s Farmers Through Big Data Sharing, AgFunder, 2016).
Open Data overlapped with Big Data could be very helpful, for example, for citizens to participate in local budgeting, choose healthcare, analyze the quality of local services, or build apps that help people navigate public transport. While considering these overlapping Open & Big data clusters, there are a few important points that should be taken into consideration:
The relation between big data and open data (The Guardian, 2014) 1. Big, Open data doesn't have to come only from government (but also, e.g., from research, social media, - to create a new, collaborative research model, to analyze public opinion etc.) | 2. Open data doesn't have to be Big Data to matter 3. Big Data that's not Open is not democratic "Big Data often tend to “cause major shifts in roles and power relations among different players in current … supply chain networks”, - Big Data in Smart Farming – A review, ScienceDirect, 2017). [NOTE: data exists as Closed, Shared or Open on a spectrum, Open Data Institute/ODI] 4. When the government turns Big Data into Open Data, it's especially powerful, for example, to the benefit of later economic growth (e.g., TheGovLab.org) |
TOWARDS THE USEFULNESS OF INTEGRATED OPEN & BIG DATA
To harness the power of Open & Big Data for (agricultural) research & development, different communities (public & private) are implementing, running and pushing their online platforms. To make an idea what these platforms are all about, you can take a look at:
Different national data.gov.XX portals, e.g., Data.gov / USA Government portal; Data.gov.au / Australian Government portal [providing link to Open Data ToolKit] ; Data.gov.za / South Africa National Data Portal; Opendata.go.tz / Tanzania Government Open Data Portal; Data.gov.uk… | DataFirst Data Portal (South Africa) |
INTEGRATION OF FAIR DATA : FROM GAPS TO SOLUTIONS
Although a great deal of effort into collecting/pushing data in/through different infrastructures is rather appealing, the field research of those same/comparable (e.g., crop research data) data and related data types around the world has remained fragmented at best.
Smooth data integration from field trials (and their data) requires support by common, shared and transparent standards, protocols, guidelines, to serve data across all (discipline-related) domains, while supporting Findable, Accessible, Interoperable and Reusable (FAIR) principles. Moreover, it is important, "that the principles apply not only to ‘data’ in the conventional sense, but also to the algorithms, tools, and workflows that led to that data". - SCIENTIFIC DATA, 2016.
Another challenge of effective data integration is connected to intellectual property rights (frequently enforced in for-profit pursuits) that weaken the proliferation of open collections and dissemination of data.
Moreover, considering that data quality assessment still remains an open issue for some organizations - - despite the area being a focal point for a number of data management professionals (Exploring open data quality, ODI, 2016) - - poor data quality could represent a further hurdle for effective data sharing and reuse.
These (and other still existing) gaps in data sharing lead often to data limitations “for all components of [agricultural] systems [and, consequently, to limitation of] knowledge products for informing decisions and policy” (Toward a new generation of agricultural system data, models, and knowledge products: State of agricultural systems science, ScienceDirect, 2016).
And that’s a problem... but ...
SOME ARE ON TO THE PROBLEM AND HAVE AN IDEA OF HOW TO FIX IT
“A systematic and better-equipped field Network can bring more scientific rigor (as well as technologies) to the field, and reduce dependence of upstream work on environmentally controlled research facilities that are unrepresentative of cropping situations”, - Improving global integration of crop research (Science, 2017).
The just quoted article discusses the idea of a central “Global Crop Improvement Network [which] would be a Central Hub where scientists could collect and analyze crop research data from around the world and apply it to create new crop varieties and develop improved practices”.
Applying open standards, recommendation, and guidelines to data integration & sharing - could be also seen as measures to improve the standardization of protocols behind global data collections (e.g., FAIRsharing, AgroPortal/e.g., CropOntology).
International communities such as ICSU World Data System, Research Data Alliancce (RDA), and Committee on Data for Science and Technology (CODATA) are continuously addressing and contributing to the thematic domains around ‘The Digital Frontiers of Global Science” (see: SciDataCon 2018), thus further helping clarify and establish some of the most important standards for each data-related under study (see, e.g., RDA Interest Groups and RDA Working Groups).
Some tools/recommendations on support of data interoperability and FAIR data sharing:
Wheat Data Interoperability Guidelines of the RDA-IGAD IG | |
Interlinking standards, repositories and data policies of the RDA-BioSharing WG |
IN SUPPORT OF DYNAMIC DATA IN DISTRIBUTED SECURE ENVIRONMENTS
Even though, a number of guidelines try to simplify the requirements for data sharing and re-usability, for data to be usable there is a lot more than it simply being technically great data.
To further unlock the value of distributed research datasets, as well as to clear competitive data concealment hurdles and the intellectual property rights, “precompetitive research” - - that implies pushing/ sharing research results/data through different (including social Web 2.0*) channels - - could be considered.
* Use of Web 2.0 Social Media Platforms to Promote Community-Engaged Research Dialogs: A Preliminary Program Evaluation (NCBI PMC) |
Different organisations are joining their forces to develop and push pre-competitive distributed secure environments
For example: |
where researchers, SMEs and large companies can share their data, collaborate on cross-partner and cross-domain challenges, through cooperation with international networks such as the European Open Science Cloud (EOSC).
EOSC : FOCUS ON FAIR & QUALITY OPEN & BIG DATA
The EOSC aims to ensure data alignment, adjust data performance indicators, and enhance data compliance with F.A.I.R. data priciples, taking into account that open data alone (even when cleaned from errors and aligned) are not enough to guarantee the quality and (re)usability of the data.
FAIR principles aims at establishing data trust based on data quality and provenance, - two important aspects that determine the fair usability of a dataset ("Published Data Objects should refer to their sources with rich enough metadata and provenance [data should be collected using reliable methods], to enable proper citation").
Trust in data implies improved data ethics. The already mentioned Open Data Institute (ODI) has been working on measures to help organisations build trust in how they collect, use and share data, and to foster better use of data overall (see: Why we need the Data Ethics Canvas).
Data Quality assessment procedures should be supported by a schema, - a set of integrity constraints and rules (Value types - Value constraints) relating to the structure and contents of a data resource (Key titles in the data).
To verify dataset’s contents, it is also useful that standards in support of data, data schema, namespaces and linked data (as a technical solution on data sharing support) are integrated in off-the-shelf data-management packages.
To check data quality, the ODI suggests to use the Open Data Certificates, which – like FAIR data principles - show the extent of the challenge of producing high quality usable data, and measure how effectively someone is sharing a dataset for ease of reuse.
The scope of the Open Data Certificates checklist covers more than just technical issues. Using best practice guidance, the Open Data Certificates checklist “mirrors” also Legal, Practical, Technical, and Social aspects related to data, thus helping assess a number of issues connected to rights and licensing, documentation, and coverage availability. A complete list of all Data Certificates is available in this Certified Datasets Registry.
According to the above envisioned scenario, producing, collecting and sharing FAIR Open & Big datasets will help further unlock competitive advantage of integrated trusted knowledge products, for the mutual benefit.
Follow #EOSC for more updates | On our way to making European Open Science Cloud reality by 2020 As to November 2017, 40 key stakeholders now endorsed European Open Science Cloud Declaration The European Commission strongly encourages you and your institution to: 1) to endorse the principles of the Declaration, and
|
Stay tuned for more news about Open & Big Data by following these (+related) hashtags on Twitter: #OpenData, #BigData, #bigdataplatform, #PUSHOpenData
Related:
OPEN DATA : Looking Back, Looking Ahead
- Open/Shared/Closed: The World of Data (ODI, video)
- Open Data in a day (ODI)
- Data Sharing is not Open Data (ODI)
- Why data-enabled insight is the key to an active nation (ODI)
- So you’ve signed the Open Data Charter, what next? (ODI)
- SPARC Europe Report : Analysis of Open Data and Open Science Policies in Europe
- Cloudy, increasingly FAIR; revisiting the FAIR Data guiding principles for the European Open Science Cloud (IOS Press)
- Big Data Trends for 2018 (DataVersity)
- How FAIR Friendly is your data catalogue? (EOSC)
- The FAIR Principles herald more open, transparent, and reusable scientific data (DTL)
- Costing Data Management (UK Data Service)
- A set of 9 Data Principles – developed by DefraDigital - you can apply to your own work
- Big Metadata : prioritizing next steps to advance Metadata Research in Data Science
- Data-cleaning toolkit: Open Refine, Drake, Data Wrangler, Data Cleaner, WinPure
- Open Data Rights Statement vocabulary (ODRS)
- Open Data Barometer (ODB) (World Wide Web Foundation)
- Data management knowledge, tools, and training (DTL)
- Donor Open Data Policy and Practice: An Analysis of Five Agriculture Programmes (GODAN)
- EUDAT B2SHARE API Presentation: How to store and publish research data using the B2SHARE API
- Strategies and guidelines for scholarly publishing of biodiversity data (RIO)
- Trusted Digital Repositories (certification)